Lesson 2: Terminology and Uncertainty in Pest Risk Analysis
Topic 2: Uncertainty in PRA
In Topic 2, you will learn about uncertainty—how it is defined and what it means—and the role that uncertainty plays in PRA. We will examine the different types of uncertainty and their sources, and we will discuss practical ways of dealing with uncertainty when conducting PRA. We will also learn the role of assumptions as we deal with uncertainty, and the importance of clearly identifying our assumptions as we draw conclusions and maintain transparency when conducting PRA.
Objectives:
- Define uncertainty
- Explain and understand the three main types and sources of uncertainty
- List and explain some practical approaches to dealing with uncertainty
- Provide examples of how and when expert judgment might be useful to reduce uncertainty in PRA
- Define assumptions and explain the term’s significance with respect to PRA
As we begin this topic, let’s recall the definition of uncertainty learned earlier. According to the Merriam-Webster online dictionary, uncertainty is something that is doubtful or unknown. As you learned in Lesson 1, uncertainty is closely linked to the concept of risk. That is, if the probability and the consequences of an adverse event are known with certainty (or are completely known), then, there is no risk.
Uncertainty and its role in risk analysis are topics with a long history in the sciences and, more recently, in the policy arena (Morgan and Henrion, 1990). Uncertainty in PRA is much more widespread than in risk analysis for food safety or animal health. Remember that in the protection of plant resources, we are dealing with thousands of potential plant pests, hundreds of potential host commodities, and an unlimited number of possible trade scenarios. Because of this, it might seem that our plant protection world is a very small island of available evidence (or information about pests, hosts, and scenarios) in a vast sea of uncertainty. Nevertheless, plant health regulatory decisions must be made.
In general, uncertainty in risk analysis can include incomplete or conflicting information, incorrect information, linguistic imprecision, bias, inappropriate methodologies, and incorrect models. In this lesson, we will examine three basic types of uncertainty:
- Insufficient information
- Variability
- Imprecision (including error)
Insufficient Information
Insufficient information is a common type of uncertainty (Yoe, 2012). It is also the easiest type to identify and understand when conducting PRA. Information gaps for a particular plant pest might include its true identity, biology, distribution, or host range. Knowledge gaps about particular phytosanitary situations or regulated articles are also commonplace.
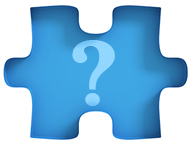
Why do we have so many gaps in our knowledge about plant pests? Considering that the universe of plant pests includes arthropods, other invertebrates (such as mollusks and nematodes), plant pathogens (such as fungi, bacteria, viruses, and others), and plants themselves (weeds), and that these plant pests can be present in any region of the world, it is not surprising that many have not been adequately studied. In some cases, we may only have a plant pest’s name, geographic location(s), and perhaps a limited description of its host range. In the case of new or undescribed species, even that level of information may not be available. Furthermore, even for plant pests that are well understood, there may still be information gaps. Other types of gaps in information might include the economic significance of pests, its hosts, or what measures, processes, or treatments are effective.
Despite all this potential for uncertainty due to insufficient information, it is important to remember that this source of uncertainty can be reduced through further study and research. Recall that under the SPS Agreement, there is no requirement for how much information is needed in order to conduct a pest risk analysis (WTO 1994). In fact, one of the benefits of conducting PRA is that it may serve to identify gaps in knowledge and instances where further research may reduce our uncertainty.
Expert judgment is a valuable resource for PRA. According to Gray et al. (1991), its use is perhaps unavoidable. After all, if we had perfect knowledge of a plant pest, we probably wouldn’t be conducting a PRA! ISPM No. 11 states that expert judgment can be used but should be clearly noted in the analysis.
Note that although expert judgment might help risk analysts in coping with uncertainty and lack of data, it might also add to the overall uncertainty in the analysis.
Variability
Variability is an unpredictable but natural variation in biological and physical processes (Yoe, 2012). Examples of variability for plant pests include level of virulence, fecundity, size, longevity, etc. Because biological organisms and systems are dynamic, variability is a ubiquitous source of uncertainty in PRA. Note that, unlike uncertainty due to insufficient information, variability cannot be reduced by gathering additional data. Nevertheless, variability may be represented more accurately and communicated more effectively with additional data. Mathematical techniques such as Monte Carlo simulation may be used to represent variability, but such analyses represent a level of sophistication that is rarely practiced in PRA.
Imprecision
Uncertainty due to imprecision comes from using incorrect or conflicting data, simplifications, approximations, or carelessness. Imprecision also results from using, making, or applying incorrect methodologies, assumptions, concepts, and terms. Imprecision also includes ambiguity or vagueness that results from misunderstandings or incorrect interpretation of language (Regan et al., 2002).
A classic example of how vagueness in the interpretation of language results in uncertainty is when we use certain words to characterize risk in PRA. In some PRA qualitative methodologies, the terms high, medium, and low are used to rate the risk of plant pests. However, failing to precisely define these terms in the context of our PRA can lead to misunderstandings in the interpretation of our PRA results and conclusions. This occurs because the terms high, medium, and low are relative terms, that is, their value is relative to some defined quantity. The uncertainty in using these relative terms is reduced by providing precise definitions of what these terms mean and specifying the context for their application in our PRA methodology.
Assumptions are very important aspects of uncertainty. The Merriam-Webster online dictionary defines assumptions as “facts or statements taken for granted.” This is an important and often overlooked problem when conducting PRA. As an analyst, it is easy to make assumptions but fail to record them in the PRA. Assumptions might not be recorded because the analyst believes they are obvious to the reader and that recording them is unnecessary. In any case, good analysts will train themselves to clearly identify the assumptions they have made in their own PRA. In other words, they will be transparent with their assumptions, while carefully searching out the assumptions in the analyses of others. A complete accounting of assumptions and a clear explanation of their rationale is essential for a true understanding of uncertainty.
Uncertainty and Risk Ratings
Although uncertainty is an essential component of risk, risk ratings in a PRA must be linked to evidence, not to uncertainty. In other words, a risk rating in a PRA should not be higher because there is high uncertainty associated with the evidence. For example, if the available evidence indicates that the risk level for a particular pest is “medium,” and the uncertainty due to lack of knowledge about that pest is high, then the risk rating for the pest should remain at “medium” with an explanation indicating that the uncertainty related to the amount or the quality of the evidence about this pest is high.
However, high uncertainty may influence judgments that are made regarding the acceptability of risk and may play a role in the initial strength of measures that are recommended to mitigate the risk. As knowledge gaps are filled and more evidence becomes available, uncertainty is reduced and judgments can be adjusted accordingly. However, it must be clear to policy makers and trading partners which aspects of the measures suggested for risk management are based on evidence and which are based on judgments regarding uncertainty.
Building confidence and predictability into pest risk analysis begins by ensuring that the process consistently handles uncertainty, however large, in ways that are predictable, scientifically defensible, and responsive to the needs of decision makers (National Research Council, 1994).
Insufficient information and variability are areas of uncertainty that a risk analyst should both identify and characterize. Analysts cannot reduce variability by gathering additional data, but they can represent it more accurately and communicate it more effectively with additional data. Imprecision is usually the result of lack of available detailed information or lack of attention to detail. To address this type of uncertainty, risk analysts should follow guidelines and templates that can assist them in the interpretation of and attention to information used in the PRA. They should also have their analyses reviewed by independent assessors (Regan et al., 2002). The more experience an analyst has with expert review, the greater the likelihood that imprecision will not be a significant factor contributing to uncertainty.
To continue, select Lesson Summary from the Topics menu above or click here.